Discover the benefits of SPSS predictive analytics for all businesses in every industry. Enhance decision-making, optimize operations, and drive growth with data-driven insights.
In today’s fast-paced and data-driven world, businesses across industries are continually looking for ways to leverage the growing volume of data at their disposal. Among the many tools available for data analysis, SPSS (Statistical Package for the Social Sciences) stands out as a powerful predictive analytics tool that has become indispensable in various fields. SPSS predictive analytics is a process that uses historical data and statistical algorithms to forecast future trends, behaviors, or outcomes, enabling businesses to make more informed decisions. The advantages of adopting SPSS predictive analytics extend across industries, from healthcare and retail to manufacturing and finance.
This paper will explore the benefits of SPSS predictive analytics for businesses in every industry, highlighting how it helps companies optimize operations, improve customer satisfaction, reduce costs, and maintain a competitive edge. By the end, it will be clear that SPSS predictive analytics is not just a tool for data scientists and statisticians but an essential business tool for organizations of all sizes.

Enhanced Decision-Making
One of the most significant advantages of SPSS predictive analytics is its ability to enhance decision-making across various levels of business operations. For businesses to remain competitive, they must be able to make accurate, timely decisions that are based on reliable data. Traditional decision-making processes often rely on intuition or limited historical insights, which can result in poor or delayed choices.
SPSS predictive analytics helps businesses overcome this challenge by enabling them to forecast future outcomes based on historical data. By using advanced statistical algorithms, companies can identify trends, patterns, and relationships within their data, which helps them anticipate future events with greater accuracy. For example, a retail business can predict future demand for a particular product, allowing it to adjust inventory levels accordingly, preventing both overstocking and stockouts.
Additionally, SPSS offers the capability to test different scenarios, helping businesses evaluate potential risks and benefits before making significant decisions. Whether a company is planning a new product launch, expanding into new markets, or restructuring its operations, predictive analytics ensures that these decisions are backed by data-driven insights rather than guesswork.
Improved Operational Efficiency
Operational efficiency is crucial for businesses looking to optimize resources, reduce costs, and improve profitability. Predictive analytics tools like SPSS enable businesses to streamline their operations by providing valuable insights into where resources should be allocated for maximum impact.
For instance, in manufacturing, SPSS predictive analytics can be used to forecast equipment failure and prevent costly downtime. By analyzing historical data on machine performance, businesses can predict when maintenance is required, thus preventing unexpected breakdowns that could disrupt the production process. Moreover, predictive analytics can help optimize supply chain management by forecasting demand fluctuations, allowing businesses to adjust production schedules and inventory levels accordingly.
In the service industry, businesses can use SPSS to predict peak times for customer traffic, enabling them to schedule staff more effectively. By ensuring that the right number of employees are available at the right time, companies can improve customer service and satisfaction while minimizing labor costs.
Increased Customer Satisfaction and Retention
Customer satisfaction and retention are paramount to the success of any business. Predicting customer behavior and preferences enables businesses to offer more personalized experiences, ultimately improving customer loyalty and retention rates.
SPSS predictive analytics allows businesses to analyze customer data, such as purchase history, browsing patterns, and social media interactions, to predict future behavior. For example, a retail company can predict which customers are most likely to make a purchase based on their past buying patterns. This insight can help the company tailor marketing efforts, offering personalized recommendations, discounts, or incentives to customers who are at risk of churn.
Moreover, predictive analytics can help businesses identify customer pain points, such as common complaints or issues with products or services. By addressing these concerns proactively, companies can enhance customer satisfaction and improve overall brand perception. This level of personalization and attentiveness can result in increased customer retention, which is often more cost-effective than acquiring new customers.

Optimizing Marketing Efforts
Effective marketing is essential for driving business growth. Traditional marketing strategies often rely on broad targeting methods, but with the help of SPSS predictive analytics, businesses can adopt a more data-driven approach that targets the right audience with the right message.
SPSS predictive analytics allows marketers to segment their customer base based on a variety of criteria, including demographics, purchasing behavior, and engagement history. By understanding the factors that drive customer decision-making, companies can develop more targeted marketing campaigns that resonate with specific groups of consumers. For instance, predictive analytics can help businesses determine the best time to reach a customer or the most effective marketing channel to use.
Additionally, SPSS can help businesses predict the effectiveness of marketing campaigns by analyzing past performance data. With this insight, companies can make adjustments to campaigns in real time, ensuring that marketing budgets are spent efficiently and that the campaigns deliver the highest return on investment (ROI).
Risk Management and Fraud Detection
Risk management is a critical concern for businesses in industries such as finance, insurance, and healthcare. SPSS predictive analytics provides valuable tools for identifying and mitigating risks, allowing businesses to make more informed decisions and avoid costly mistakes.
In the financial industry, for example, predictive analytics can be used to detect fraudulent activities by analyzing transaction patterns and identifying anomalies. By identifying suspicious behavior early, businesses can take proactive measures to prevent fraud and minimize financial losses. This is especially valuable in sectors such as banking, where fraud detection is a top priority.
In addition to fraud detection, SPSS predictive analytics can help businesses assess and mitigate various types of risk, including credit risk, operational risk, and market risk. By analyzing historical data and identifying potential risk factors, businesses can develop strategies to manage or reduce exposure to these risks.
Competitive Advantage
The ability to predict future trends and behaviors provides businesses with a significant competitive advantage. Companies that leverage predictive analytics can stay ahead of their competitors by anticipating market shifts, customer preferences, and industry trends.
For example, a retail business that uses SPSS predictive analytics to forecast consumer demand can adjust its inventory levels and pricing strategies in advance, ensuring that it is well-positioned to meet customer needs before its competitors. Similarly, companies in the tech industry can use predictive analytics to forecast the success of new product releases, allowing them to time their product launches more effectively and capture market share.
Furthermore, businesses that adopt predictive analytics can identify emerging opportunities and potential threats that may otherwise go unnoticed. By using data-driven insights to guide decision-making, companies can stay agile and adapt to changing market conditions more quickly than their competitors.
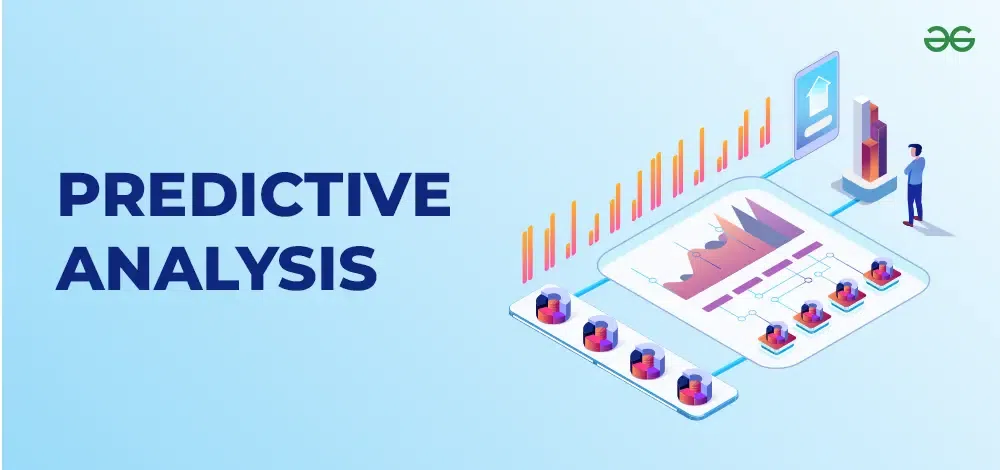
Better Resource Allocation
One of the challenges faced by businesses of all sizes is efficiently allocating resources. Whether it’s financial resources, human capital, or physical assets, businesses must ensure that they are using their resources in the most effective way possible to maximize returns.
SPSS predictive analytics can help businesses identify which areas of their operations are underperforming and which are thriving. This allows them to allocate resources more effectively, prioritizing areas that offer the highest return on investment. For example, predictive analytics can help businesses identify high-performing products, services, or customer segments, allowing them to focus their efforts on these areas.
Moreover, predictive analytics can optimize labor management by forecasting staffing needs based on customer demand and workload projections. In industries such as retail and hospitality, this can result in a more efficient workforce and better overall performance.
Scalability and Flexibility
SPSS predictive analytics is a scalable solution that can be adapted to businesses of all sizes. Whether a small startup or a large multinational corporation, SPSS offers flexible tools that can be tailored to meet the specific needs of each organization. Businesses can start with basic predictive models and gradually incorporate more advanced techniques as their data analysis needs evolve.
As businesses grow and accumulate more data, SPSS can scale to handle large volumes of information without compromising on performance. This scalability ensures that businesses can continue to leverage predictive analytics as they expand, making it an invaluable long-term investment.
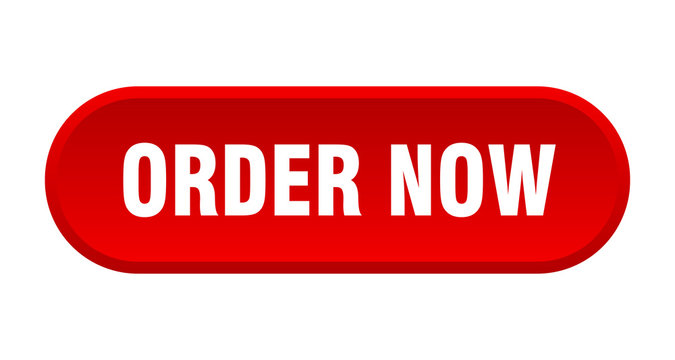
Conclusion
SPSS predictive analytics offers a wealth of benefits for businesses across every industry, ranging from improved decision-making and operational efficiency to enhanced customer satisfaction and competitive advantage. By harnessing the power of data, businesses can make more informed decisions, mitigate risks, and optimize resources, all of which contribute to long-term success and profitability.
In an increasingly data-driven world, businesses that fail to adopt predictive analytics risk falling behind their competitors. By embracing tools like SPSS, companies can stay ahead of the curve, making smarter, more strategic decisions that enable them to thrive in their respective industries. SPSS predictive analytics is not just a tool for the data scientist but an essential business solution that helps organizations unlock the full potential of their data and drive meaningful growth.