Collaborative Work Using SPSS enhances data analysis efficiency and accuracy. Discover strategies for sharing projects, integrating insights, and working seamlessly with teams on SPSS. Statistical Package for the Social Sciences (SPSS) is one of the most widely used software tools for statistical analysis in research across various disciplines such as psychology, sociology, health sciences, and business. SPSS offers a powerful platform to analyze data and draw conclusions based on empirical evidence. One of the key advantages of using SPSS is the ability to facilitate collaborative work among researchers, statisticians, and analysts. Collaborative work allows teams to leverage the collective expertise of multiple individuals, leading to more accurate, comprehensive, and insightful data analysis.
This paper explores the concept of collaborative work using SPSS, highlighting its advantages, challenges, and examples of successful collaborations in various research projects. In addition, the paper will discuss the integration of online SPSS tools and the increasing trend of remote collaborative data analysis.

The Importance of Collaborative Work in Data Analysis
Collaborative work is crucial in research and data analysis, particularly when complex datasets are involved. SPSS, as a versatile tool for statistical analysis, can greatly benefit from teamwork because:
Specialized Expertise
Different team members can bring their own specialized knowledge to the table. For example, a team might consist of a subject matter expert, a statistician, and a data scientist. Each member can contribute their expertise to ensure the most robust data analysis possible.
Error Reduction
Working collaboratively reduces the chances of errors. When multiple people are reviewing and analyzing the data, the likelihood of missing or misinterpreting critical insights is diminished. Peer review is an essential aspect of any research process.
Time Efficiency
By dividing tasks such as data cleaning, variable coding, hypothesis testing, and interpretation, teams can complete a data analysis project in a shorter period. This is particularly advantageous in situations where time is of the essence, such as during grant applications or conference presentations.
Better Decision-Making
Collaborative teams can often come to more balanced and accurate conclusions by pooling together their various perspectives. With diverse expertise, teams can approach problems from different angles and propose more reliable solutions.
In the context of SPSS, collaboration can occur both in person and remotely, with the software facilitating data sharing, analysis, and visualization across different platforms.
The Role of SPSS in Collaborative Data Analysis
SPSS is designed with a user-friendly interface that simplifies complex statistical analysis. The software includes a wide range of functions, such as descriptive statistics, regression analysis, factor analysis, and more, making it an ideal choice for collaborative work. The software’s features can be maximized when teams engage in collaborative work.
Key Features of SPSS for Collaborative Work
Multiple File Formats
SPSS allows for data storage in different formats, including .sav, .csv, and .xls, making it easier for team members to share datasets and analyses. This flexibility ensures that different members of the team, regardless of their preferred software, can access and contribute to the project.
Syntax and Output Sharing
SPSS enables researchers to save and share syntax (the commands that tell the software how to perform tasks), as well as output files. The ability to store and share syntax means that team members can easily replicate analyses, thereby ensuring consistency across different stages of the analysis.
Graphical Capabilities
SPSS comes with robust graphing tools that are essential for visualizing data in a collaborative project. These graphical outputs help teams better understand trends, patterns, and relationships within the data. Furthermore, these visuals are easily shareable, promoting collaboration and further analysis.
Built-in Collaboration Tools
SPSS offers functionalities for collaboration through cloud-based storage, such as the IBM SPSS Statistics Collaboration feature. With this tool, teams can work simultaneously on the same dataset, share analyses, and track changes made by various team members.
Version Control
SPSS supports version control, allowing teams to keep track of the various iterations of their analysis. This is especially useful for larger projects where different team members work on different sections, as it ensures that the latest version of the analysis is always accessible.
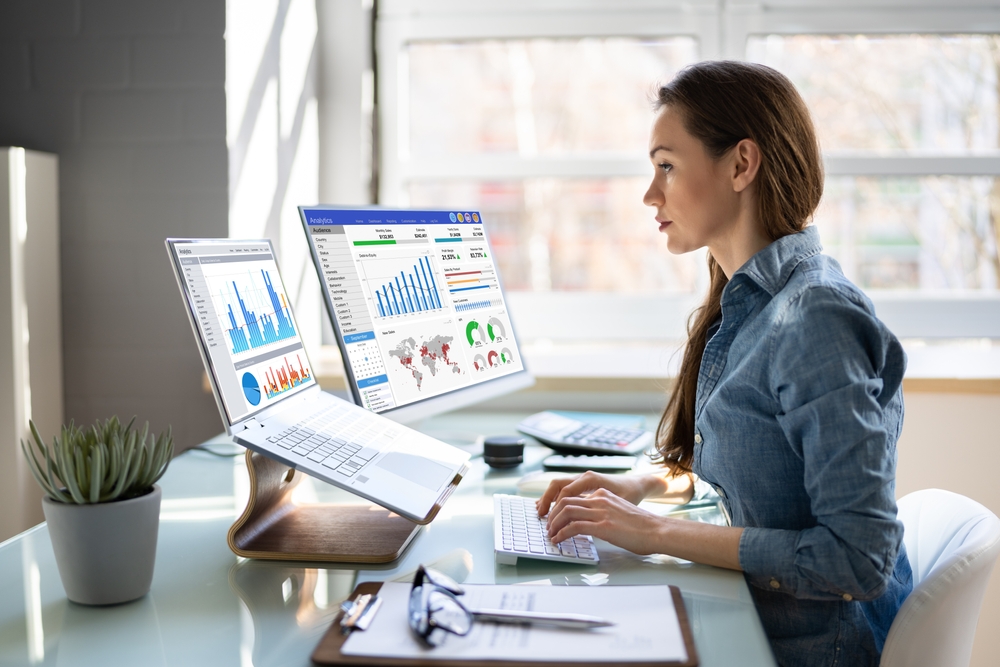
Collaborative Work Using SPSS: Example of a Research Project
A practical example of collaborative work using SPSS can be seen in the field of social sciences. Suppose a team of researchers is investigating the impact of social media use on mental health among teenagers. The team consists of three individuals:
- Dr. Smith: A subject matter expert with extensive experience in adolescent psychology.
- Dr. Johnson: A statistician with expertise in analyzing large-scale datasets.
- Sarah: A graduate student working on the project and skilled in using SPSS for data cleaning and preliminary analysis.
The project begins with Dr. Smith designing a survey to measure various variables such as frequency of social media use, levels of anxiety, and self-esteem among teenage participants. The raw data from the survey is then provided to Sarah, who uses SPSS to clean the dataset by checking for missing values, outliers, and inconsistencies.
Once the data is cleaned, Sarah shares the dataset and her preliminary analysis (including descriptive statistics) with Dr. Johnson, who uses advanced statistical techniques like regression analysis to assess the relationships between social media use and mental health outcomes.
Throughout the project, the team uses SPSS’s collaboration features to share syntax files and output files, ensuring that each team member is working with the most up-to-date information. They also use SPSS’s graphical tools to create visual representations of their findings, which are later included in the research paper.
This example highlights how collaborative work using SPSS can result in a more comprehensive and accurate analysis, as each member brings their unique expertise to the project. The ability to share files, track changes, and collaborate in real time ensures that the research process is streamlined and efficient.
Online SPSS Data Analysis: The Future of Collaboration
With the advent of cloud computing and remote collaboration tools, online SPSS platforms have become increasingly popular. These platforms offer a range of benefits that can enhance collaborative work, especially when team members are located in different geographical locations.
Benefits of Online SPSS for Collaborative Work
Real-Time Collaboration
Online platforms such as IBM SPSS Statistics Subscription allow team members to work on the same dataset simultaneously. This real-time collaboration eliminates the need for back-and-forth file sharing, making the process faster and more efficient.
Accessibility
Online SPSS platforms are accessible from any device with an internet connection, allowing team members to work from anywhere. This flexibility is particularly beneficial for global teams or those working remotely.
Integration with Other Tools
Many online SPSS platforms integrate with other collaboration tools, such as Google Drive and Dropbox. This enables seamless file sharing and communication between team members.
Cost-Effectiveness
Subscription-based online SPSS platforms often offer more affordable options than purchasing a full license of the software. This makes it more accessible for smaller research teams, educational institutions, or independent researchers.
Automatic Updates
Online SPSS platforms are regularly updated, ensuring that users have access to the latest features, bug fixes, and security patches. This ensures that teams are always working with the most up-to-date version of the software.
Example of Online Collaborative Data Analysis Using SPSS
Consider a team of researchers spread across different time zones who are investigating the relationship between employee engagement and job satisfaction. By using an online SPSS platform, the team can divide tasks based on time zone availability. For example, a researcher in Europe can clean the data, while a researcher in the United States can run regression analyses, and a third researcher in Asia can create visualizations.
In addition, the team can collaborate on a shared document, discussing their analysis and interpretations in real time. Using online SPSS tools, they can also integrate data from external sources and analyze large datasets more efficiently.
This example underscores the efficiency and effectiveness of online SPSS platforms for collaborative work, particularly in a globalized research environment.
Challenges of Collaborative Work Using SPSS
Despite the many advantages, collaborative work using SPSS does come with its challenges. Some of the common obstacles include:
Data Privacy and Security
Sharing sensitive data through cloud-based platforms can raise concerns about privacy and security. Teams must take appropriate measures, such as data encryption and secure file sharing, to protect confidential information.
Software Compatibility
Team members may be using different versions of SPSS, which can lead to compatibility issues. Ensuring that everyone is working with the same version of the software is crucial to avoid discrepancies in the analysis.
Communication Barriers
While SPSS’s collaboration features can streamline the analysis process, effective communication among team members is still essential. Misunderstandings or misinterpretations of data can occur, especially if there is a lack of clear communication or regular meetings.
Learning Curve
Not all team members may be familiar with SPSS, particularly those with limited experience in statistical analysis. This may require additional training or support to ensure that everyone can contribute effectively.
Conclusion
Collaborative work using SPSS offers numerous benefits, from enhanced accuracy and efficiency in data analysis to the pooling of expertise from multiple team members. Whether in person or online, SPSS provides an ideal platform for teamwork, allowing researchers to analyze complex datasets and draw meaningful conclusions.
With the rise of online SPSS platforms, remote collaboration has become more accessible and efficient than ever before. While challenges remain, such as data security concerns and software compatibility issues, the advantages of collaborative data analysis far outweigh the obstacles.
As research teams continue to embrace SPSS for data analysis, the future of collaborative work in this field looks promising. By leveraging the power of teamwork and the capabilities of SPSS, researchers can achieve more comprehensive, insightful, and impactful results.